AI today is considered to be one of the most groundbreaking technologies that carry the potential of changing multiple industries, amplifying human abilities, and resolving numerous challenges facing the world today.
Based on the existing data some trends can be predicted for development of artificial intelligence (AI) during the years 2024 such as the problems connected with privacy and data protection, ethically questionable such as the problem of algorithmic bias or lack of transparency of the methods used, and socio-economic such as the consequences of automation and introduction of new technologies leading to job displacement. To tackle these issues, there is a need to integrate effort from different fields and put effort to enforce regulations policies.
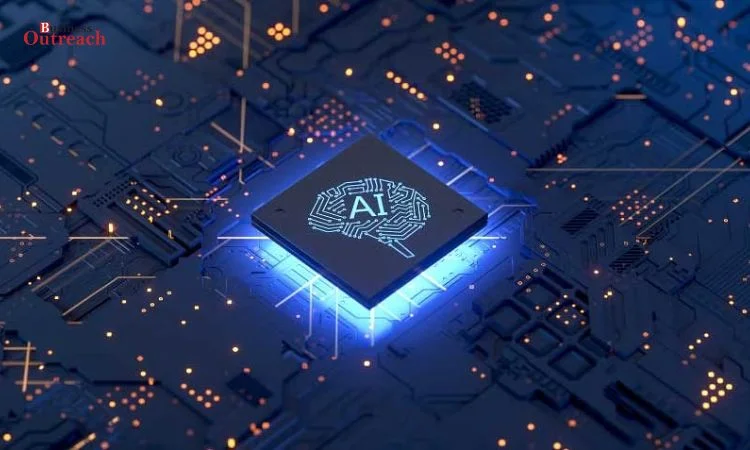
Source: Simplilearn.com
The broad integrations of AI are also seen as symbols of potential threats to cybersecurity, especially when implementing an international and ethical approach to this issue. Similarly, it also means that while the potential of AI is inarguably immense, a great deal of care needs to be taken to ensure that the technology is used for the betterment of society, and that the advantages thus generated are not overshadowed by its disadvantages.
Here Below Are The 15 Challenges Of Artificial Intelligence(AI)
Challenge | Description |
AI Ethical Issues | Issues related to privacy infringement, reinforcement of prejudice, and social responsibilities. Bias in algorithms leads to discriminatory outcomes. |
Bias in AI | Biases in training data are magnified, leading to unfair outcomes, such as discrimination in employment, credit, and legal penalties. |
AI Integration | Challenges in applying AI to manufacturing and service delivery, including data compatibility and organizational culture. |
Computing Power | Requires highly efficient computing hardware, with issues related to cost, energy efficiency, and hardware architecture. |
Data Privacy and Security | Protection of data from unauthorized access, loss, and tampering. Differential privacy and federated learning are essential. |
Legal Issues with AI | Liability, ownership of digital assets, and regulation of AI-generated content. Judicial responsibility in decision-making and system failures. |
AI Transparency | Need for explainability and transparency in AI models to ensure reliability and user confidence. |
Building Trust | Transparency and accountability are crucial for user acceptance of AI systems. |
Lack of AI Explainability | Difficulty in understanding AI decision-making processes, leading to loss of trust. |
Discrimination | AI systems can amplify societal biases, affecting areas like job finding and credit applications. |
Limited Knowledge of AI | Lack of awareness among decision-makers, adopters, and regulators about AI’s potential and limitations. |
High Expectations | AI’s potential leads to high expectations, but it has deficiencies and challenges. |
Implementation Strategies | Requires methods for integrating AI into organizational structures and operations. |
Data Confidentiality | Protection of personal information from unauthorized access and disclosure. |
Software Malfunction | AI software can produce wrong outputs, system crashes, and be vulnerable to attacks. |
AI Ethical Issues
Ethics in AI refers to a topic of discussion that comprehensively involves aspects such as privacy infringement, reinforcement of prejudice, and social responsibilities. The issues emerging now are all linked to the ability to guarantee non-discriminatory, explainable and transparent artificial intelligence decision-making. Bias – which in many algorithms manifest in discriminating against a certain group- is one of the most dangerous threats the internet poses by deepening inequalities.
This means that leaders should approach the use of AI particularly in fields that raise much concern such as health and law enforcement in a more targeted manner. That is why there is a greater need to pay most attention to ethical considerations while struggling for success in these fields. Finding the middle ground between the former and the latter is key to using AI to augment, rather than erode, the human experience: to optimise the potential of pattern matching for the general good, whilst minimising the dangers of a ‘black box’ approach to information processing.
Bias in AI
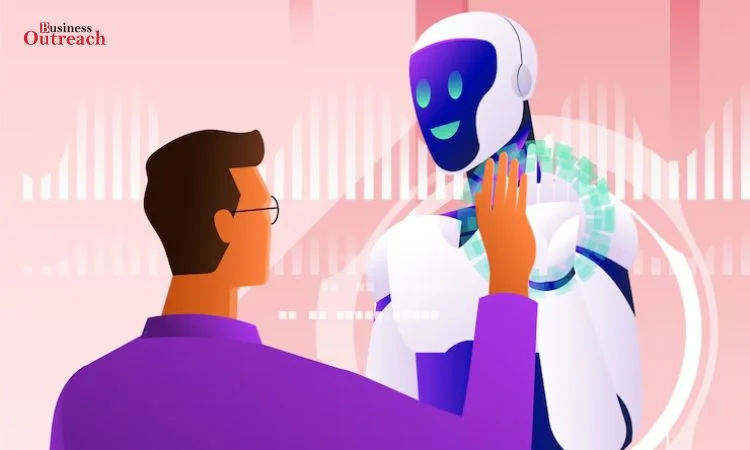
It suggests that when creating machine learning algorithms many biases that are already apparent in the training process are reinvented and magnified. This can happen sometimes and leads to unfair and unfair results that are unethical at the same time and make part of disadvantaged groups suffer the most.
Some examples include cases of discrimination in employment where employees of certain groups are hired while those of others are not, unfair credit granting where some groups are granted loans while others are not, and unjust penalties where some criminals are given harsher penalties than others with similar crimes.
Reducing AI bias should be made in a systematic method that entails selecting and processing a specific type of data, employing specific preprocessing techniques and developing right and appropriate algorithms. BIAS detection and prevention are critical, and this means conducting periodic and cross-system analyses to determine instances of AI bias in decision-making.
AI Integration
Applying artificial intelligence to manufacturing and service delivery to accurately implement the new changes that strive to improve automation. In this process, reference application scenarios are identified, the AI models are adapted to the different application areas, and compatibility with existing systems are checked. Positive integration requires effective partnership that involves the IT specialists in AI and the professionals in relevant business fields in order to arrive at specific solutions for organizations.
Data compatibility, people issues, and organizational culture. These challenges need to be solved through more planning, communication, and incrementalism regarding the AI features and improving them while avoiding interference and disruption with others. A successful Artificial Intelligence integration can lead to a remarkable shift in paradigms and serve as a potentiation tool in all spheres of business.
Computing Power
Flexibility of computing resources is vital for the creation of AI models, especially those who entail complex computations and subsequently, large datasets. AI requires highly efficient computing hardware such as GPUs and TPUs and the demand increases with the advancement of rich algorithms.
Some of the current critical issues associated with this area are the cost, energy efficiency, and has-a-size-that-is-proportional-to-the-key properties of these systems. Solutions with regard to the fundamental aspects of hardware architecture like neuromorphic computing and quantum computing are also a part of early-stage developments.
Lastly, distributed computing and cloud services can increase the process computational capabilities for overcoming the limitations of computation. Appealing for more computational resources in the development and operation of AI, however, needs to be coordinated with the efficiency and cost of meeting those demands.
Data Privacy and Security
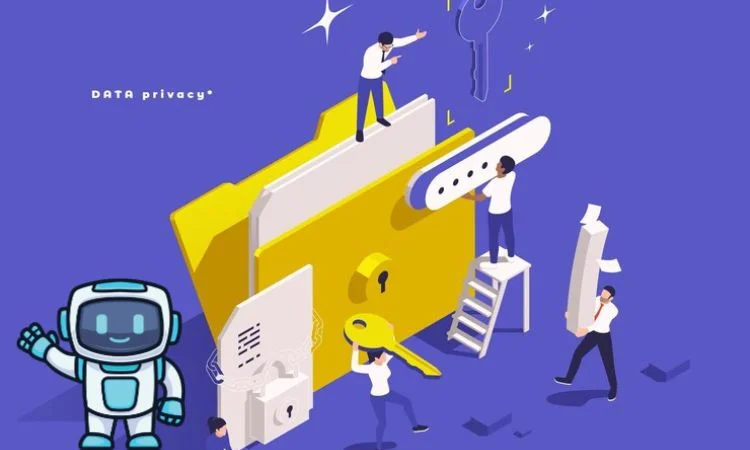
Security of data and data privacy are critical ever increasing concerns in AI as the large volume of data is a key to the functioning and learning of AI systems. The utmost importance of protecting data from unauthorized access, loss and tampering is a mandatory security requirement.
Any organization needs to adhere to the above regulations through safeguard measures like; restrictions on access, encryption methods, and audit mechanisms.
In the same way, there’s also a need to introduce methods such as differential privacy and federated learning into the process to minimise privacy risks without compromising the usefulness of the outcomes. It is valuable to establish trust among users by providing proper data processing processes and acceptable data control measures that makes the AI systems safe and improves data usage.
Legal Issues with AI
It equally poses a number of legal issues mainly on liability, ownership of digital assets, and regulating consumption of virtual projected materials. Judicial responsibility emerges as a crucial issue in situations where AI is a part of decision-making and during AI system failure or accident occurrences involving AI autonomous systems.
Some potential problems that relate to AI are legal matters of authorship and ownership of a piece of content that is produced by an artificial intelligence system or an algorithm.
Playing the utmost attention to anti-legal risks seems appropriate as the development of regulations lags behind technological advancements. Solving these problems implies a coordination effort taking place between legal scholars, lawmakers and computer scientists for producing intelligible rules and standards that promote technological advancement and innovation while, at the same time, ensuring that fair and adequate responsibility frameworks are in place for sensitive justice protection of stakeholders.
AI Transparency
AI explainability is the process of explaining the rationale of the AI techniques used to develop or implement an AI system. It is vital for stating its reliability, and inherent accountability as well as user confidence. Transparency talks about the comprehensiveness of certain models and structures such as AI and its parts, sources, results, and processes. Bridging techniques like explainable AI (XAI) helps in offering robust interpretations of the AI models to enhance the comprehensibility of AI systems.
In addition, documenting data sources, the training process of the model, and algorithms used to estimate performance also elaborates on the subject. The implementation of transparency helps organizations and companies to show how they are using AI in a responsible and fair manner, reduce instances of bias and give their users the chance to have control over the given results from the AI systems.
Building Trust
It is important to note that trust is a factor that plays an important role in acceptance of AI systems, which we need to address. The basis of this trust is the compliance with principles such as the disclosure of information, the provision of services, and the assumption of responsibility. Transparency is another concern in AI, where organizations need to show users how the AI system is operating and the logic used in making decisions.
This element refers to the ability to carry out the task as designed many times to produce standard results such as correct outputs. In today’s paper accountability is seen as the process of acknowledging the responsibility of the outcomes produced by the AI and the handling of any mistakes or bias produced by the AI system.
Lack of AI Explainability
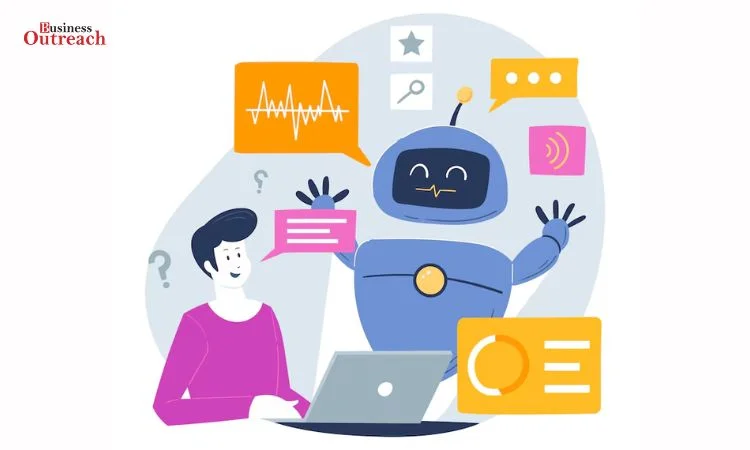
AI(artificial intelligence) opacity is a form of opacity which is a condition that explicitly acknowledges that it is hard to understand how an AI decided on a certain solution. This lack of transparency can result in a loss of trust or even in industries where it is a vital factor, such as health care and money related fields.
This, however, is a problem that is in the process of being solved by employing AI methodologies as means of getting a glimpse into the decision making process of an AI algorithm. Utility tools including feature importance analysis and model visualisation are especially useful for users to better understand AI outputs. Nonetheless, there is still the problem of achieving explainability while not losing the ability to develop high-performance models.
Discrimination
AI bias refers to unfair treatment by the artificial intelligence systems that provide short ends of the stick to certain people or groups based on factors such as colour or gender. One of the biggest risks of using AI systems is that the biases are baked into the training data and so the injustice that exists in society is merely amplified. For example, such algorithms concretely influence people’s lives when finding a job or applying for credit and can reinforce existing disparities.
To combat this discrimination, there is the need to address the bias in data collection and in algorithms. Other existing paradigms, like fairness-aware machine learning, attempt to make the world more fair by addressing the issue at the model-building stage. Due to its principles of fairness and non-bias, AI systems are capable of identifying and eliminating unfair treatment of applicants hence protecting the rights of everyone.
Limited Knowledge of AI
However, decision-makers, adopters, and regulators remain limited in their knowledge regarding AI and its potential due to lack of awareness. This lack of knowledge results in misapprehensions and misstatements of AI’s potentials and restrictions, thus hampering the proper application of its potential and expansion.
To this end, the best solution lies in the need to establish proper educational programs, as well as public awareness initiatives, which will allow the society to get a clearer understanding of the principles and applications of artificial intelligence and the possible effects it may produce.
Moreover, access to helpful tools and training could also encourage people to use AI effectively in their day-to-day lives. Tackling the knowledge deficit requires interdisciplinary cooperation, engaging local stakeholders, and social contributions. This approach will make it possible for society to harness the best out of the emerging applications of AI while at the same time looking at the darker side of the new developments.
High Expectations
One of the key advantages of AI is the ability to achieve high levels of performance, which causes high expectations . Despite these promising features, AI has its deficiencies and challenges most of the time to cover its potential hype.
Public awareness has been noted to be another major area where these stakeholders require to be educated on the strengths of AI and the areas where it can be of more relevance. Implementable goals and raising awareness of potential and limitations are the fundamental keys to ensure organizations get optimistic realities and do not get disappointed to harness AI.
Implementation Strategies
Significant deployment of AI requires methods whereby these innovations can be incorporated into structures and operations.
practices that allow for the adoption of the AI technologies into the systems of an organization or a business.
Key aspects include:
1. Selecting Proper Use Cases: Leaving the question of the general tendencies in AI applications and their directions for future development aside, let us focus on the possibilities of linking the application of artificial intelligence to quite specific organizational and strategic goals.
2. Evaluating Data Quality: This means that in data collection the researcher has to make sure that data is adequate and of good quality.
3. Choosing Suitable AI Models: Some of the important steps that the software should be able to do well include selecting the right artificial intelligence algorithms or models.
Moreover, establishing an innovation advisory board will foster the necessary experimentation and learning essential for incrementally building and improving the AI applications you pursue. Domain knowledge in integrations of domain experts and AI experts in a team is contemporarily incorporating AI solutions into context to fit user requirements while also considering the organization requirements.
Data Confidentiality
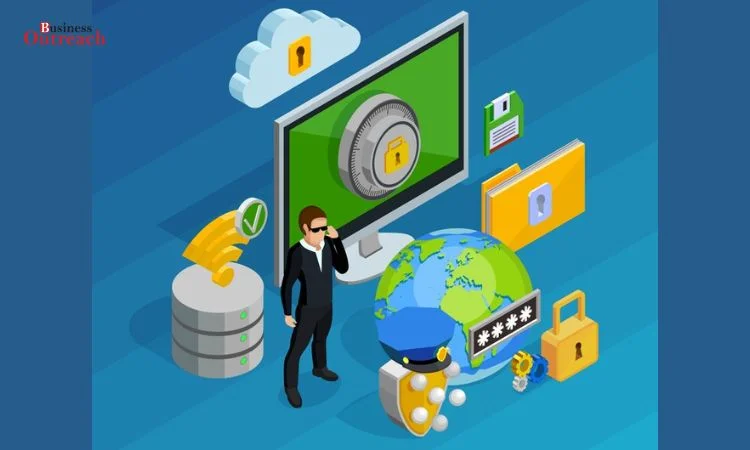
The preservation of data confidentiality is crucial in AI since it protects the personal information shared from being seen and disclosed by those who have no right to do so. Even when data is created, committed, manipulated, stored and disposed there is need for tight security measures that include encryption, access control and storage security measures.
Therefore, the GDPR and HIPAA act as legal formalities in protecting individual proprietary information and form the basis for ethical handling of data. Privacy considerations are one of the key factors that users and stakeholders rely on to approve and invest in AI solutions, which is why privacy protection is so important to the development of good, reliable and responsible AI.
Software Malfunction
There are corresponding threats associated with the use of AI software such as the capability of the AI producing wrong outputs, the possibility of system crashes, and vulnerability of attacks. Therefore, adequate measures need to be taken in order to reduce these risks and this include testing and quality assurance procedures which must be implemented throughout the lifecycle of the software.
Additionally, creating an ecosystem promoting such practices increases the chances of identifying defects in the software and eradicating them, leading to safer and more dependable AI systems.
Conclusion
AI today is considered one of the most groundbreaking technologies, carrying the potential to transform multiple industries, amplify human abilities, and resolve numerous global challenges. However, this immense potential is accompanied by significant challenges that need to be addressed for AI to be truly beneficial.